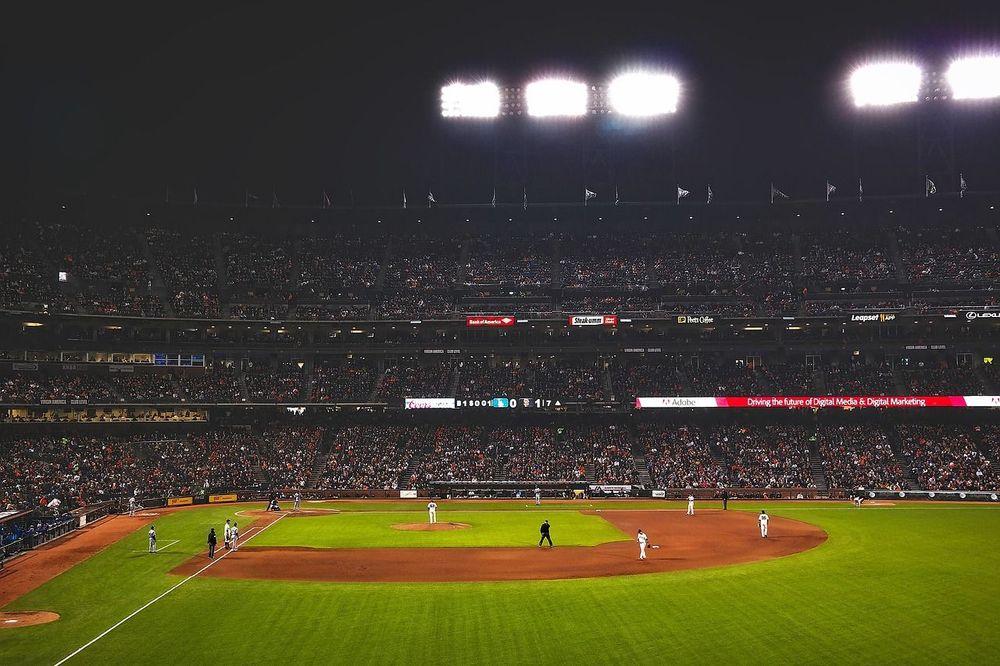
Offseason recap: Reviewing our final scorecard, and trends we saw
Due to the baseball stoppage amidst the global pandemic, the 2020 baseball season hasn’t yet begun, which means that we’re still, awkwardly, in offseason mode. It’s like the party guest who wouldn’t leave at 2 am.
But: It’s given us a bit of a breather here at BTV to do some catch-up work and reflect on how our model performed during the offseason, and to re-test it based on some new inputs. Here’s an update on all of that.
Testing our model
First, how did our model perform relative to all real-life trades this offseason? We’ll look at it two ways: first, as measured at the time of each trade, when our model was reflecting only partial data; second, as measured when our data set was complete:
With partial data:
Total trades: 45
Total accepted by our system: 37
Percentage accepted: 82.2%
Average variance: 4.6
Not bad. But we then re-ran the trades using more complete data:
With completed data:
Total trades: 45
Total accepted by our system: 41
Percentage accepted: 91.1%
Average variance: 1.9
Much better. But how do we explain the difference in accuracy? Let’s start with the nature of player valuation updates.
On the major-league side, to find the closest correlations to real life, we use a composite weighting of various publicly available WAR projections. This varies by position. The model is at its most accurate when all of those numbers are complete.
However, the reality is that, throughout the course of the offseason, we are dealing with incomplete data. We start with our own projections (using the Marcel method, which gets us in the ballpark), then incorporate data from the other major public systems as it comes in. Because those systems publish in dribs and drabs, often late in the offseason, our values are continuously changing to reflect each new data point.
Similarly, on the minor league side, we use a weighted composite of the most respected prospect evaluators. But here again, we have to wait for them to publish (either their team lists or Top 100 lists), at which point we jump on them and translate their ratings into valuations according to our model. So if you see a swing on a certain prospect, that’s because one of those outlets changed their rating on that player. Typically, though, it’s not a dramatic swing because our model is stable enough (and updated frequently enough) to incorporate these in moderation.
So what this means is that we have two different timeframes with which to judge the accuracy of our model: at the time of the trade (with incomplete data and estimates) and after the trade (with complete data, backtested).
The fact that we see strongly correlated numbers after all data is in (91.1% accepted, with a 1.9 variance) tells us that our model is robust and fairly accurate.
The flip side of that is that the model will be a little less accurate when real-life trades occur in November, December and January.
Adjustments to our model
The offseason offers a unique opportunity to test our model against market free-agent market pricing. After all, in theory a free agent’s salary should roughly match his field value, netting out at roughly zero surplus value. However, it’s not that simple, because supply-and-demand factors influence the prices, which leads to big contracts for some types of players (such as high-quality starting pitchers) and small contracts for others (such as aging second basemen). Those market forces inform our trade value estimates as well.
We did make some subtle changes to our model based on two interesting trends we saw consistently:
Offense vs. defense: We knew that the market valued bats more than gloves, but this year the differences were stark. Nick Castellanos (great bat, but a butcher in the field) got a four-year, $64M deal; Yolmer Sanchez (gold glove defender, but a below-average bat) had to settle for a minor-league deal.
Related to this point is that good hitters are harder to find than good defenders. Scarcity matters. This is also true among individual positions where defense matters (like catchers and shortstops).
Aces vs. back-end starters. Scarcity also matters with pitchers. This is likely because there is more marginal win value with aces -- contending teams will pay up for a Gerrit Cole or a Stephen Strasburg because the wins they produce are more valuable to them than to a mediocre or losing team. They are also factoring in post-season contributions. Good starting pitching is rare, and therefore expensive (either in free agency or the trade market); mediocre starting pitching is abundant, and therefore cheap (in both markets). So even an injury-prone Hyun-Jin Ryu can get a nice four-year deal, but the Jhoulys Chacins and Trevor Cahills settle for minor-league deals if at all.
The point is that this offseason confirmed what we already knew: that the market value of WAR contributions is variable. The scarcity of high-WAR players skews their valuations higher, and the abundance of low-end players skews their valuations lower. This was only somewhat factored into our calculations previously, but now you’ll find it applied more broadly.